Table Of Content
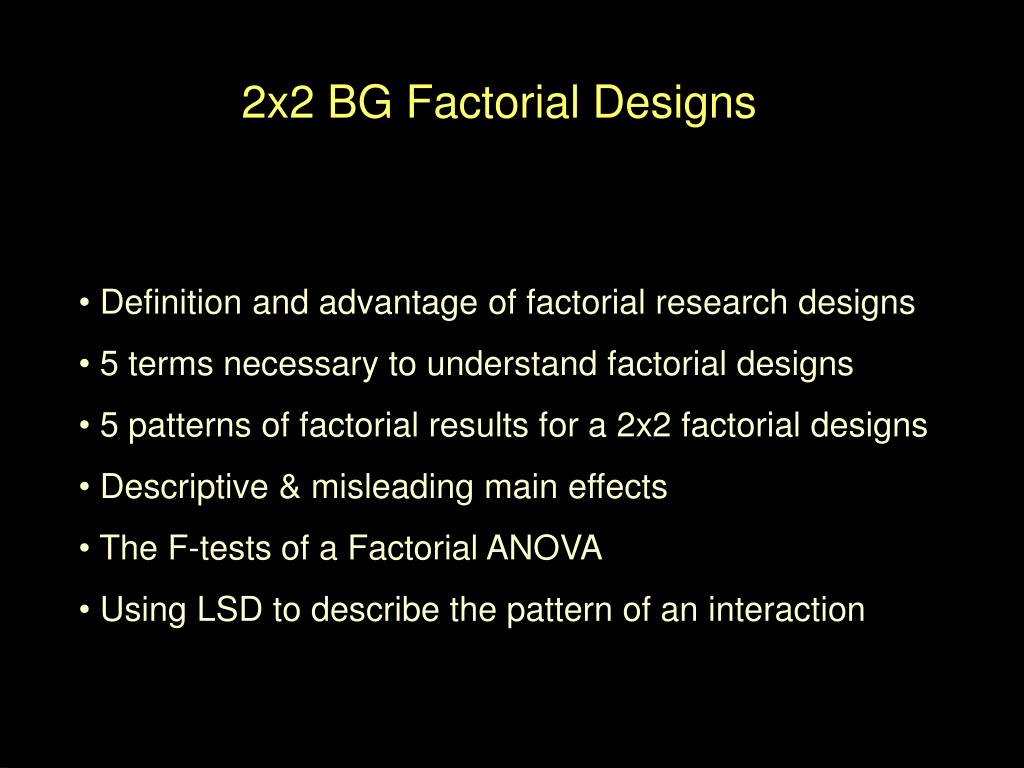
In the bottom panel, independent variable “B” again has an effect at both levels of independent variable “A”, but the effects are in opposite directions. One example of a crossover interaction comes from a study by Kathy Gilliland on the effect of caffeine on the verbal test scores of introverts and extraverts [Gil80]. Introverts perform better than extraverts when they have not ingested any caffeine. But extraverts perform better than introverts when they have ingested 4 mg of caffeine per kilogram of body weight. It would seem almost wasteful to measure a single dependent variable. A full factorial design may also be called a fully crossed design.
Excel: How to Highlight Entire Row Based on Cell Value
Other options can be selected from the "Analyze Factorial Design" menu such as "Covariates...", "Prediction...", "Storage...", and "Weights...". Consult the "Help" menu for descriptions of the other options. Once all desired changes have been made, click "OK" to perform the analysis. All of the plots will pop-up on the screen and a text file of the results will be generated in the session file. The only option in this menu is the number of replicates to add. To have a total of 3 trials of each, the user should add 2 replicates in this menu.
Factorial ANOVA
For example, subject 1 had a 10 and 5 in the no-reward condition, so their mean is 7.5. Just looking at the means, we can see there was a main effect of Distraction, the mean for the no-distraction condition was 11.1, and the mean for the distraction condition was 6.8. The size of the main effect was 4.3 (the difference between 11.1 and 6.8). The overall means for for each subject, for the two distraction conditions are shown to the right. For example, subject 1 had a 10 and 12 in the no-distraction condition, so their mean is 11. We could say the same thing, but talk from the point of view of the second IV.
Paired Samples t-test: Definition, Formula, and Example
The researcher would consider the main effect of sex, the main effect of self-esteem, and the interaction between these two independent variables. Thus far we have seen that factorial experiments can include manipulated independent variables or a combination of manipulated and non-manipulated independent variables. But factorial designs can also include only non-manipulated independent variables, in which case they are no longer experiment designs, but are instead non-experimental in nature. This can be conceptualized as a 2 × 2 factorial design with mood (positive vs. negative) and self-esteem (high vs. low) as non-manipulated between-subjects factors. In many factorial designs, one of the independent variables is a non-manipulated independent variable. One independent variable was disgust, which the researchers manipulated by testing participants in a clean room or a messy room.
Randomized Phase II Study of All-Trans Retinoic Acid and Valproic Acid Added to Decitabine in Newly Diagnosed ... - ScienceDirect.com
Randomized Phase II Study of All-Trans Retinoic Acid and Valproic Acid Added to Decitabine in Newly Diagnosed ....
Posted: Fri, 24 Dec 2021 06:31:19 GMT [source]
In these cases, it is common to only run a single replicate of the design, and to assume that factor interactions of more than a certain order (say, between three or more factors) are negligible. Under this assumption, estimates of such high order interactions are estimates of an exact zero, thus really an estimate of experimental error. Again, the main effect of posture was not the primary effect of interest.
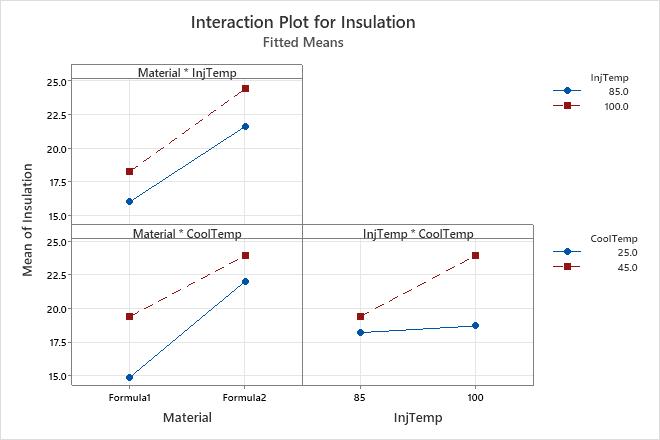
Population vs. Sample: What’s the Difference?
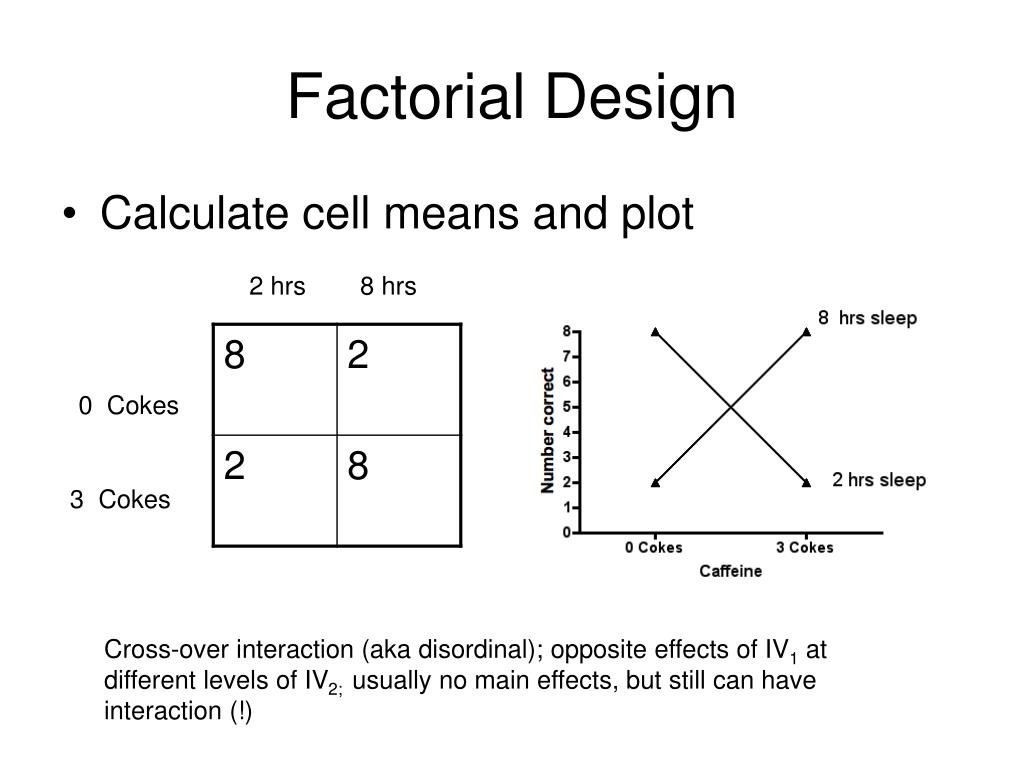
Although this might seem complicated, you already have an intuitive understanding of interactions. It probably would not surprise you, for example, to hear that the effect of receiving psychotherapy is stronger among people who are highly motivated to change than among people who are not motivated to change. This is an interaction because the effect of one independent variable (whether or not one receives psychotherapy) depends on the level of another (motivation to change). If they were high in private body consciousness, then those in the messy room made harsher judgments. If they were low in private body consciousness, then whether the room was clean or messy did not matter.
Analysis
We could have one IV (coffee), with three levels (1, 2, or 3 coffees). You might want to say we have three manipulations here, drinking 1, 2, or 3 coffees. Nevertheless, we say you are only doing one coffee manipulation.
Factorial Designs¶
An example is a study by Halle Brown and colleagues in which participants were exposed to several words that they were later asked to recall (Brown, Kosslyn, Delamater, Fama, & Barsky, 1999)[1]. Some were negative health-related words (e.g., tumor, coronary), and others were not health related (e.g., election, geometry). Since factorial designs have more than one independent variable, it is also possible to manipulate one independent variable between subjects and another within subjects.
Modifying DOE Table
To illustrate, a 3 x 3 design has two independent variables, each with three levels, while a 2 x 2 x 2 design has three independent variables, each with two levels. It is also possible to manipulate one independent variable between subjects and another within subjects. But he or she might choose to treat time of day as a between-subjects factor by testing each participant either during the day or during the night (perhaps because this only requires them to come in for testing once). Factorial design is an important method to determine the effects of multiple variables on a response. Traditionally, experiments are designed to determine the effect of ONE variable upon ONE response.
In the table, a yes means that there was statistically significant difference for one of the main effects or interaction, and a no means that there was not a statisically significant difference. As you can see, just by adding one more independent variable, the number of possible outcomes quickly become more complicated. When you conduct a 2x2 design, the task for analysis is to determine which of the 8 possibilites occured, and then explain the patterns for each of the effects that occurred. There is an interaction effect (or just “interaction”) when the effect of one independent variable depends on the level of another.
In the other condition, people will get no money, but they will still have find differences. Remember, this will be a factorial design, so everybody will have to find differences when they are distracted and when they are not distracted. However, we can also perform a two-way ANOVA to formally test whether or not the independent variables have a statistically significant relationship with the dependent variable. Plotting the means is a visualize way to inspect the effects that the independent variables have on the dependent variable. Suppose that you are looking to study the effects of hours slept (A), hours spent with significant other (B), and hours spent studying (C) on a students exam scores.
PEACE-1: Upfront triplet therapy gives OS advantage to men with mCSPC - Medicine Matters oncology
PEACE-1: Upfront triplet therapy gives OS advantage to men with mCSPC.
Posted: Mon, 20 Sep 2021 07:00:00 GMT [source]
Once the terms have been chosen, the next step is determining which graphs should be created. The types of graphs can be selected by clicking on "Graphs..." in the main "Analyze Factorial Design" menu. In the "Modify Design" menu, users can modify factors, replicate design, randomize design, renumber design, fold design, and add axial points. Additionally, any changes made can be put into a new worksheet. To change the factors, click the "Modify factors" radio button and then "Specify" to see the following options menu. Before the participant arrives, randomly organize packets with each of the four combinations of conditions to ensure that group assignments are entirely based on chance.
Generally a researcher measures an effect of interest (their IV 1). Then, they want to know what makes that effect get bigger or smaller. They want to exert experimental control over their effect. For example, they might have a theory that says doing X should make the effect bigger, but doing Y should make it smaller. They can test these theories using factorial designs, and manipulating X or Y as a second independent variable. What if you wanted to manipulate more than one independent variable?
No comments:
Post a Comment